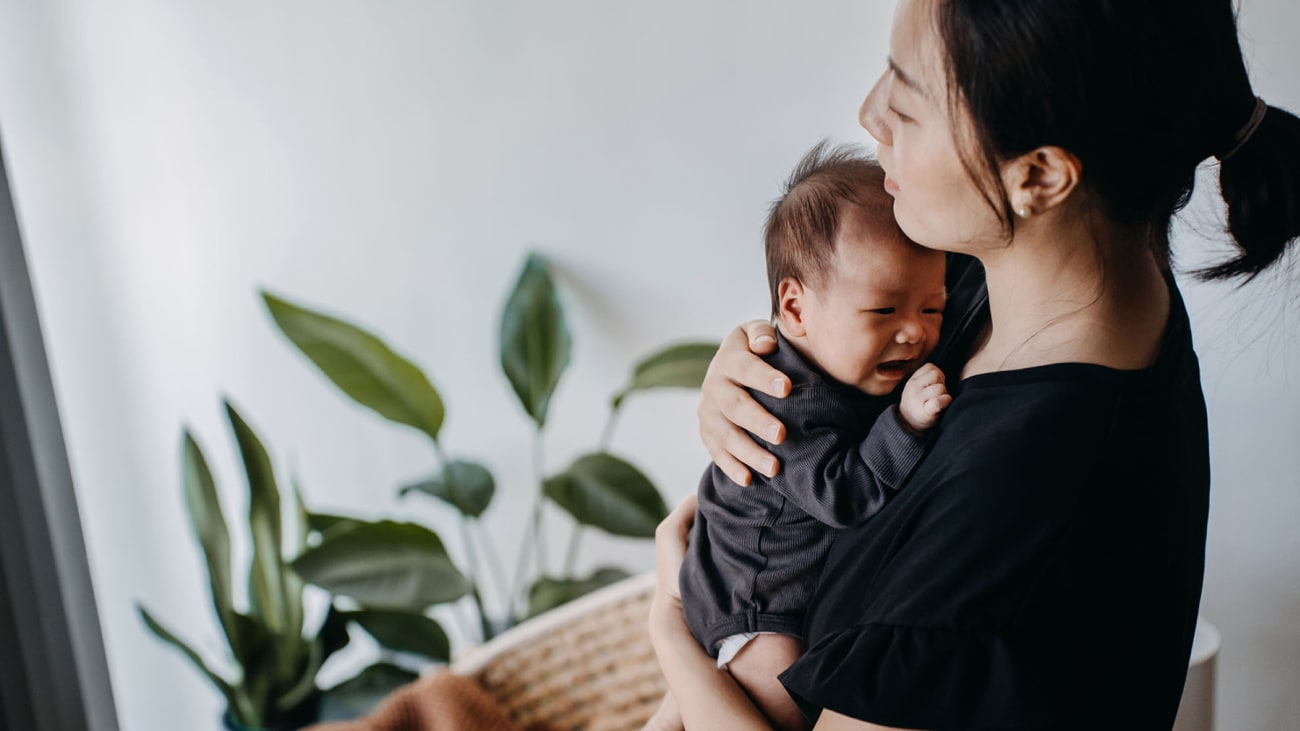
Cedars-Sinai Investigators Use Electronic Health Records to Assess Risk Without Perpetuating Racial Biases
Cedars-Sinai investigators have created machine learning (ML) models, a type of artificial intelligence, that can use patients’ electronic health records to predict—independent of racial minority biases—the risk of postpartum depression.
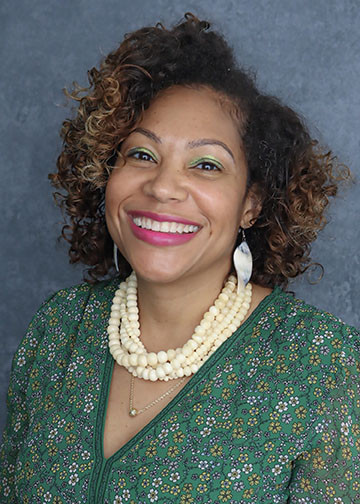
Tiffani J. Bright, PhD
The ML models, described in the peer-reviewed journal JAMA Network Open, were more likely to correctly identify postpartum depression in racial minorities compared to non-Hispanic white patients. Because many postpartum women who are Black or Hispanic are not properly diagnosed with postpartum depression, the use of these models could help improve early detection across all racial groups, potentially leading to more equitable healthcare outcomes.
“Prior models assumed that all women evaluated for postpartum depression received the same treatment and often did not account for racial disparities,” said Tiffani J. Bright, PhD, senior author of the study and the co-director of the Center for Artificial Intelligence Research and Education and research assistant professor in the Department of Computational Biomedicine at Cedars-Sinai. “We were able to create models that did not perpetuate the same biases often seen in prior prediction models, suggesting the critical role of equity-centered modeling decisions in ensuring equitable patient care.”
Additional Cedars-Sinai authors include Emily F. Wong, PhD; Anil K. Saini, PhD; Eynav E. Accortt, PhD; Melissa S. Wong, MD; and Jason H. Moore, PhD.
Funding: This research was supported by NIH National Center for Advancing Translational Science (UCLA CTSI Grant Number UL1TR001881), which supports Cedars-Sinai’s Honest Enterprise Research Broker (HERB) Committee that is responsible for extracting data from electronic health records for research purposes. We would also like to thank the departments of Obstetrics & Gynecology, Psychiatry, Nursing, and Case Management, and the Nursing Healthcare Teams for implementing the Quality Improvement project. The first and corresponding author, Emily F. Wong, had full access to all the data extracted for this study and takes responsibility for the integrity of the data and the accuracy of the data analysis.
Follow Cedars-Sinai Academic Medicine on LinkedIn for more on the latest basic science and clinical research from Cedars-Sinai.